Why Predictive Analytics Has Finally Arrived for Renewables
May 1, 2018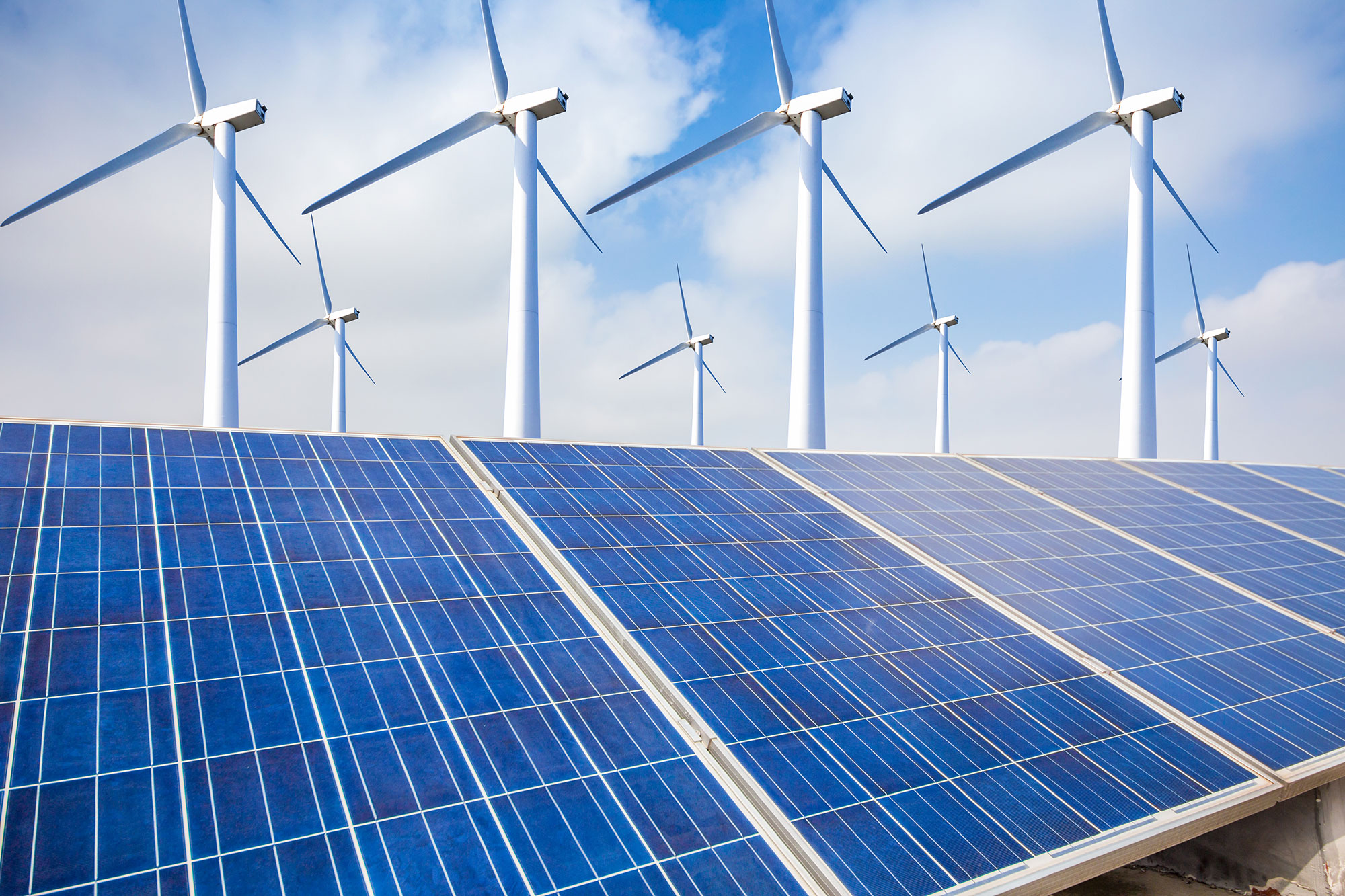
Up to now the renewable energy industry has used data chiefly as a monitoring tool. Gearboxes in wind turbines equipped with sensors report back vibrational and efficiency data. Solar mirror or solar photovoltaic (PV) farms might relay back real-time position and movement as panels follow the sun.
But the real payoff in the data access world comes from predicting total power output and payback analysis before the actual project even gets off the ground.
This means investors who are financing the project will want to see “proof of payback” by running compound models on the “levelized cost of energy” (LCOE), efficiencies based on historical weather data, regulatory impediments and many other key performance indicators (KPIs).
Until recently complex modeling might only be attainable via manual work by a team of engineers. But today there are cloud-based Web apps that can achieve the same results through a model orchestration process.
Imagine a developer of a wind farm approaches several banks to finance a large project they have in mind for a specific tract of land in Texas. The argument must be made that this will be a “slam dunk” for the investor — that is, they will get their money back with a healthy profit margin based on the best estimates of the wind farm’s performance and cost. With the right inputs, a cloud-based app can produce a reasonably accurate model-based prediction on whether the wind farm project will get built and operate efficiently enough to see a good return. This will be based on pre-populated inputs based on geography, historical weather data, wind speed and direction, and even turbine gearbox failure rates.
Combining orchestration of performance and economic models is one important way to get developers and investors in renewable projects on the same page.